AI in Pharma: Promise vs. Reality - Bridging the Gap
The pharmaceutical industry stands on the brink of an AI revolution, yet a significant gap exists between potential and practical application. Discover why embracing AI fully is crucial for the future of pharmaceuticals.
Artificial Intelligence is reshaping the pharmaceutical landscape, offering unprecedented opportunities for innovation and efficiency. In drug discovery, AI algorithms analyze vast datasets of chemical compounds and biological processes, significantly accelerating the identification of potential drug candidates. This technology has reduced the time and cost associated with traditional drug development methods, which typically span 15 years and cost billions of dollars. AI's impact extends beyond drug discovery. In clinical trials, machine learning algorithms optimize patient selection, predict drug interactions, and improve success rates. Personalized medicine has also seen advancements, with AI analyzing patient data to tailor treatments to individual genetic profiles. Furthermore, AI enhances operational efficiency in manufacturing processes and supply chain management.
The potential of AI in pharmaceuticals is vast. From generating new molecules with desired properties to synthesizing biological sequences and simulating complex clinical scenarios, AI is driving innovation across the entire drug development pipeline. This technological revolution promises to deliver faster, safer, and more cost-effective drug development processes, ultimately benefiting patients worldwide.
The Reality Check: AI Adoption Challenges
Despite the promising potential of AI in pharmaceuticals, the industry faces significant challenges in its widespread adoption and practical implementation. While many companies claim to use AI, the reality is that deep, transformative AI integration remains elusive for most.
One of the primary obstacles is the lack of suitable IT infrastructure. Many pharmaceutical companies operate on legacy systems not designed for AI integration, necessitating substantial investments in upgrading their technological capabilities. This misalignment between existing infrastructure and AI requirements has slowed adoption rates considerably.
Another critical challenge is the shortage of AI expertise within pharmaceutical organizations. The complex nature of AI technologies requires specialized knowledge that many companies lack in-house. This knowledge gap has created a disconnect between AI's potential and its practical application in day-to-day operations.
Data quality and accessibility also pose significant hurdles. AI algorithms require vast amounts of high-quality, well-structured data to function effectively. However, many pharmaceutical companies struggle with data silos, inconsistent data formats, and privacy concerns, limiting the effectiveness of AI applications.
Regulatory uncertainty further complicates AI adoption. The lack of clear guidelines for validating and approving AI-driven drug development processes has led to hesitancy among industry players, particularly in areas directly impacting patient safety and drug efficacy.
These challenges have resulted in a significant gap between AI's theoretical potential and its practical implementation. Industry experts estimate that only about 30% of pharmaceutical companies have successfully integrated AI into their core processes, with even fewer achieving transformative results from their AI initiatives.
A Framework for Deep AI Integration
To bridge the gap between AI's promise and its practical application in pharmaceuticals, a strategic framework is essential. This approach should focus on three key areas: the "Why," the "How," and the "What" of AI integration.
Why: Understanding the Value Proposition
Companies must clearly articulate why AI is crucial for their specific needs. This involves:
Identifying key pain points in current processes
Quantifying potential benefits in terms of time, cost, and innovation
Aligning AI initiatives with overall business strategy
How: Building the Foundation
Successful AI integration requires:
Investing in robust, AI-compatible IT infrastructure
Developing in-house AI expertise through training and strategic hiring
Establishing data governance protocols to ensure high-quality, accessible data
Collaborating with regulatory bodies to navigate compliance challenges
What: Implementing Targeted AI Solutions
Focus on:
Prioritizing AI projects with the highest potential impact
Starting with pilot programs to demonstrate value and gain organizational buy-in
Continuously measuring and communicating AI-driven outcomes
Iterating and scaling successful AI applications across the organization
By adopting this framework, pharmaceutical companies can move beyond surface-level AI applications and unlock its transformative potential. Deep AI integration requires commitment, investment, and a cultural shift towards data-driven decision-making. However, the rewards – in terms of accelerated drug discovery, improved patient outcomes, and enhanced operational efficiency – make this journey essential for the future of the pharmaceutical industry.
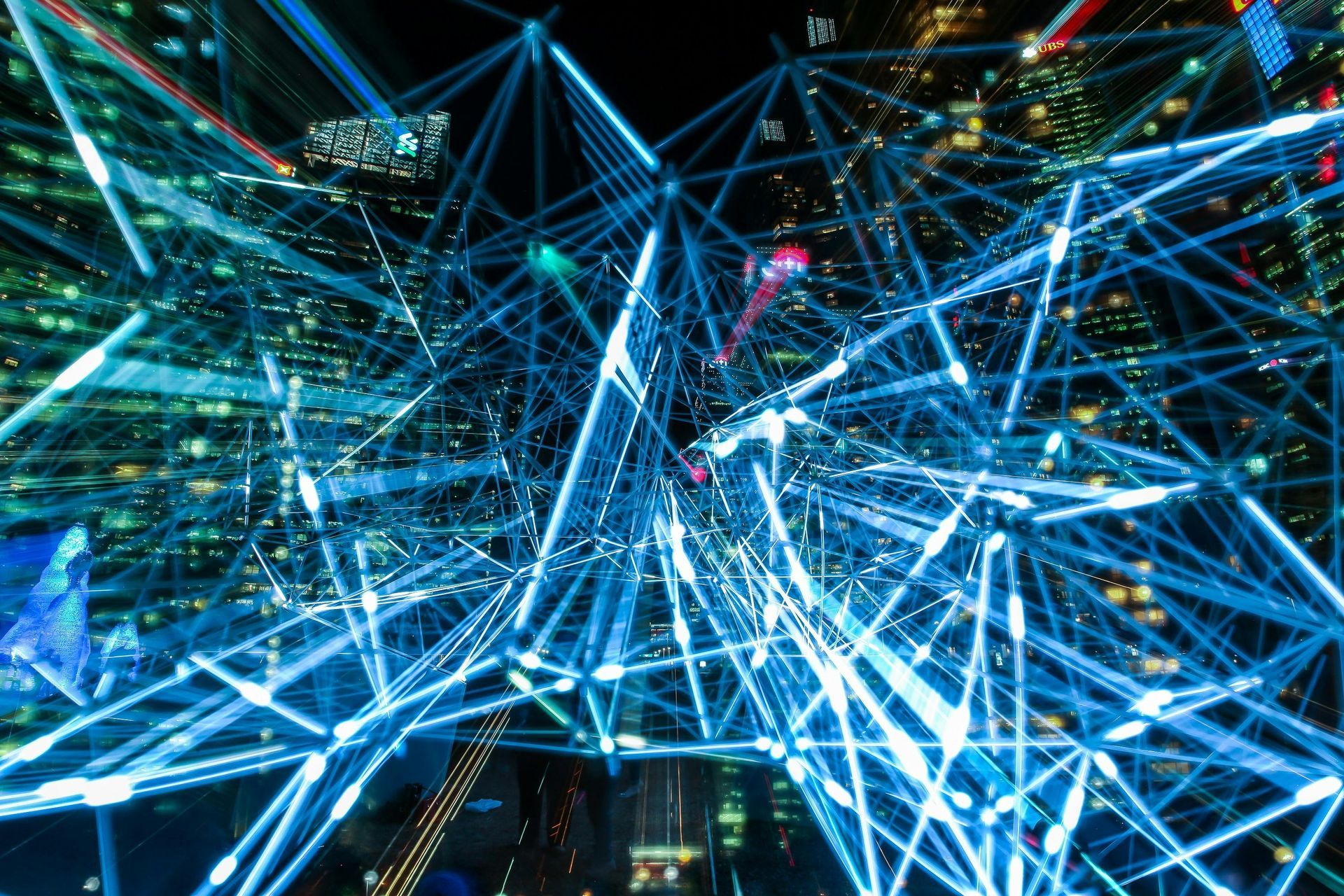